CATS: Difference between revisions
Jump to navigation
Jump to search
No edit summary |
|||
(13 intermediate revisions by the same user not shown) | |||
Line 3: | Line 3: | ||
R package for power estimation for a two-stage genome-wide association design. This is a modification of the code from Skol et al 2006, nat genet. so that the relative risk, case-control ratios and allele frequencies are allowed to vary between stages. | R package for power estimation for a two-stage genome-wide association design. This is a modification of the code from Skol et al 2006, nat genet. so that the relative risk, case-control ratios and allele frequencies are allowed to vary between stages. | ||
= cite = | |||
<pre> | |||
Skol, AD, Scott, LJ, Abecasis, GR, Boehnke, M (2006). Joint analysis is more efficient than replication-based analysis for two-stage genome-wide association studies. Nat. Genet., 38, 2:209-13. | |||
</pre> | |||
= Download and installation = | = Download and installation = | ||
[http://popgen.dk/software/download/CATS/CATS_1. | This package only seems to work on linux. | ||
[http://popgen.dk/software/download/CATS/CATS_1.02.tar.gz Software version 1.02] | |||
[http://popgen.dk/software/download/CATS/CATS-manual.pdf manual] | [http://popgen.dk/software/download/CATS/CATS-manual.pdf manual] | ||
install R package from command line | |||
<pre> | |||
wget http://popgen.dk/software/download/CATS/CATS_1.02.tar.gz | |||
R CMD INSTALL CATS_1.02.tar.gz | |||
</pre> | |||
= run in R = | |||
<pre> | |||
library(CATS) | |||
cats(freq=0.2,ncases=500,ncases2=500,ncontrols=1000,ncontrols2=1000,risk=1.5,multiplicative=1) | |||
Expected Power is; | |||
For a one-stage study = 0.94 | |||
For first stage in two-stage study = 0.972 | |||
For second stage in replication analysis = 0.784 | |||
For second stage in a joint analysis = 0.929 | |||
pi = 0.5 | |||
</pre> | |||
= plot examples = | = plot examples = | ||
=== | ==== Which design is better ==== | ||
[[File:catsFig1.png|thumb]] | |||
<pre> | <pre> | ||
library(CATS) | library(CATS) | ||
rr<-seq(1,2,by=0.05) | rr<-seq(1,2,by=0.05) | ||
maf<-c(0.05,0.1,0.2,0.5) | maf<-c(0.05,0.1,0.2,0.5) | ||
c2<-curve.cats(rr,maf,ncases=600,ncontrols=600,ncases2=600,ncontrols2=600,alpha=0.000001,prevalence=0.01); | c2<-curve.cats(rr,maf,ncases=600,ncontrols=600,ncases2=600, | ||
ncontrols2=600,alpha=0.000001,prevalence=0.01); | |||
plot(c2,type="One",main="power2",ylab="Power",xlab="RR",file=NULL,col=1:4) | plot(c2,type="One",main="power2",ylab="Power",xlab="RR",file=NULL,col=1:4) | ||
Line 24: | Line 55: | ||
lines.cats(c2,type="First",lty=4) | lines.cats(c2,type="First",lty=4) | ||
legend("left",c("One stage","Joint","Relication","First Stage"),lty=1:4,bty="n") | legend("left",c("One stage","Joint","Relication","First Stage"),lty=1:4,bty="n") | ||
</pre> | |||
==== Number of SNPs for replication ==== | |||
[[File:catsFig2.png|thumb]] | |||
<pre> | |||
library(CATS) | |||
power.J96<-c() | |||
power.J1536<-c() | |||
RR<-seq(1.1,1.5,by=0.025) | |||
maf=c(5,10,20,50)/100 | |||
for(tal2 in 1:length(maf)){ | |||
J1<-c() | |||
J2<-c() | |||
for(tal in 1:length(RR)){ | |||
temp<-cats(freq=maf[tal2],ncases=1500,ncontrols=1500,ncases2=2000, | |||
ncontrols2=2000,risk=RR[tal],pimarkers = 96/300000,alpha=0.05/300000) | |||
J1[tal]<-temp$P.joint | |||
temp<-cats(freq=maf[tal2],ncases=1500,ncontrols=1500,ncases2=2000, | |||
ncontrols2=2000,risk=RR[tal],pimarkers = 1536/300000,alpha=0.05/300000) | |||
J2[tal]<-temp$P.joint | |||
} | |||
power.J96<-cbind(power.J96,J1) | |||
power.J1536<-cbind(power.J1536,J2) | |||
} | |||
col=1:length(maf) | |||
plot(RR,power.J1536[,1],type=type,lwd=2,ylab="Power",main="Multiplicative model, | |||
1500:1500, 2000:2000",col=col[1],ylim=0:1) | |||
for(tal2 in 2:length(maf)){ | |||
lines(RR,power.J1536[,tal2],lwd=2,col=col[tal2],type=type) | |||
} | |||
for(tal2 in 1:length(maf)){ | |||
lines(RR,power.J96[,tal2],lwd=2,col=col[tal2],type=type,lty=2) | |||
} | |||
legend("bottomright",c(paste("MAF=",c(maf),", rep=1536"),paste("MAF=",c(maf),", rep=96")),col=col,lwd=2,bty="n",lty=c(rep(1,length(maf)),rep(2,length(maf)))) | |||
</pre> | |||
==== heatmap of power ==== | |||
[[File:catsFig3.png|thumb]] | |||
<pre> | |||
library(CATS) | |||
rr<-seq(1,2,by=0.025) | |||
c<-super.cats(rr,by=length(rr),ncases=765,ncontrols=1274,ncases2=100,ncontrols2=100, | |||
alpha=0.001,prevalence=0.01); | |||
plot(c,main="power",file=NULL) | |||
</pre> | |||
==== design and MAF ==== | |||
[[File:catsFig4.png|thumb]] | |||
<pre> | |||
library(CATS) | |||
rr<-seq(1,2,by=0.05) | |||
maf<-c(0.05,0.1,0.2,0.5) | |||
c2<-curve.cats(rr,maf,ncases=600,ncontrols=600,ncases2=600,ncontrols2=600, | |||
alpha=0.000001,prevalence=0.01); | |||
plot(c2,type="One",main="power2",ylab="Power",xlab="RR",file=NULL,col=1:4) | |||
lines.cats(c2,type="Replication",lty=3) | |||
lines.cats(c2,type="Joint",lty=2) | |||
lines.cats(c2,type="First",lty=4) | |||
legend("left",c("One stage","Joint","Relication","First Stage"),lty=1:4,bty="n") | |||
</pre> | </pre> |
Latest revision as of 09:28, 4 September 2017
joint power of a non-symetric two stage GWA design
R package for power estimation for a two-stage genome-wide association design. This is a modification of the code from Skol et al 2006, nat genet. so that the relative risk, case-control ratios and allele frequencies are allowed to vary between stages.
cite
Skol, AD, Scott, LJ, Abecasis, GR, Boehnke, M (2006). Joint analysis is more efficient than replication-based analysis for two-stage genome-wide association studies. Nat. Genet., 38, 2:209-13.
Download and installation
This package only seems to work on linux.
install R package from command line
wget http://popgen.dk/software/download/CATS/CATS_1.02.tar.gz R CMD INSTALL CATS_1.02.tar.gz
run in R
library(CATS) cats(freq=0.2,ncases=500,ncases2=500,ncontrols=1000,ncontrols2=1000,risk=1.5,multiplicative=1) Expected Power is; For a one-stage study = 0.94 For first stage in two-stage study = 0.972 For second stage in replication analysis = 0.784 For second stage in a joint analysis = 0.929 pi = 0.5
plot examples
Which design is better
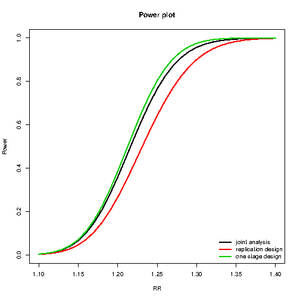
library(CATS) rr<-seq(1,2,by=0.05) maf<-c(0.05,0.1,0.2,0.5) c2<-curve.cats(rr,maf,ncases=600,ncontrols=600,ncases2=600, ncontrols2=600,alpha=0.000001,prevalence=0.01); plot(c2,type="One",main="power2",ylab="Power",xlab="RR",file=NULL,col=1:4) lines.cats(c2,type="Replication",lty=3) lines.cats(c2,type="Joint",lty=2) lines.cats(c2,type="First",lty=4) legend("left",c("One stage","Joint","Relication","First Stage"),lty=1:4,bty="n")
Number of SNPs for replication
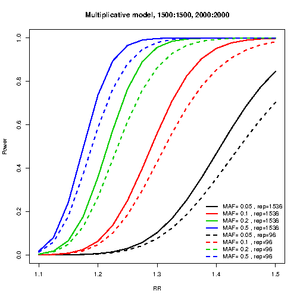
library(CATS) power.J96<-c() power.J1536<-c() RR<-seq(1.1,1.5,by=0.025) maf=c(5,10,20,50)/100 for(tal2 in 1:length(maf)){ J1<-c() J2<-c() for(tal in 1:length(RR)){ temp<-cats(freq=maf[tal2],ncases=1500,ncontrols=1500,ncases2=2000, ncontrols2=2000,risk=RR[tal],pimarkers = 96/300000,alpha=0.05/300000) J1[tal]<-temp$P.joint temp<-cats(freq=maf[tal2],ncases=1500,ncontrols=1500,ncases2=2000, ncontrols2=2000,risk=RR[tal],pimarkers = 1536/300000,alpha=0.05/300000) J2[tal]<-temp$P.joint } power.J96<-cbind(power.J96,J1) power.J1536<-cbind(power.J1536,J2) } col=1:length(maf) plot(RR,power.J1536[,1],type=type,lwd=2,ylab="Power",main="Multiplicative model, 1500:1500, 2000:2000",col=col[1],ylim=0:1) for(tal2 in 2:length(maf)){ lines(RR,power.J1536[,tal2],lwd=2,col=col[tal2],type=type) } for(tal2 in 1:length(maf)){ lines(RR,power.J96[,tal2],lwd=2,col=col[tal2],type=type,lty=2) } legend("bottomright",c(paste("MAF=",c(maf),", rep=1536"),paste("MAF=",c(maf),", rep=96")),col=col,lwd=2,bty="n",lty=c(rep(1,length(maf)),rep(2,length(maf))))
heatmap of power
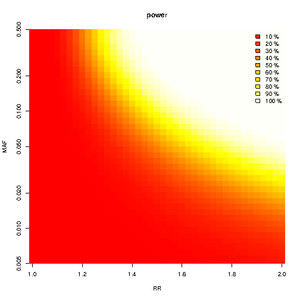
library(CATS) rr<-seq(1,2,by=0.025) c<-super.cats(rr,by=length(rr),ncases=765,ncontrols=1274,ncases2=100,ncontrols2=100, alpha=0.001,prevalence=0.01); plot(c,main="power",file=NULL)
design and MAF
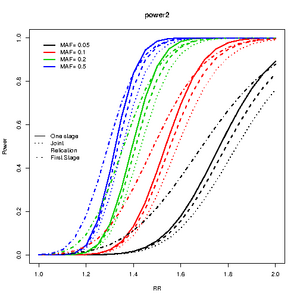
library(CATS) rr<-seq(1,2,by=0.05) maf<-c(0.05,0.1,0.2,0.5) c2<-curve.cats(rr,maf,ncases=600,ncontrols=600,ncases2=600,ncontrols2=600, alpha=0.000001,prevalence=0.01); plot(c2,type="One",main="power2",ylab="Power",xlab="RR",file=NULL,col=1:4) lines.cats(c2,type="Replication",lty=3) lines.cats(c2,type="Joint",lty=2) lines.cats(c2,type="First",lty=4) legend("left",c("One stage","Joint","Relication","First Stage"),lty=1:4,bty="n")